A Comprehensive Workflow for Skill Identification and Management
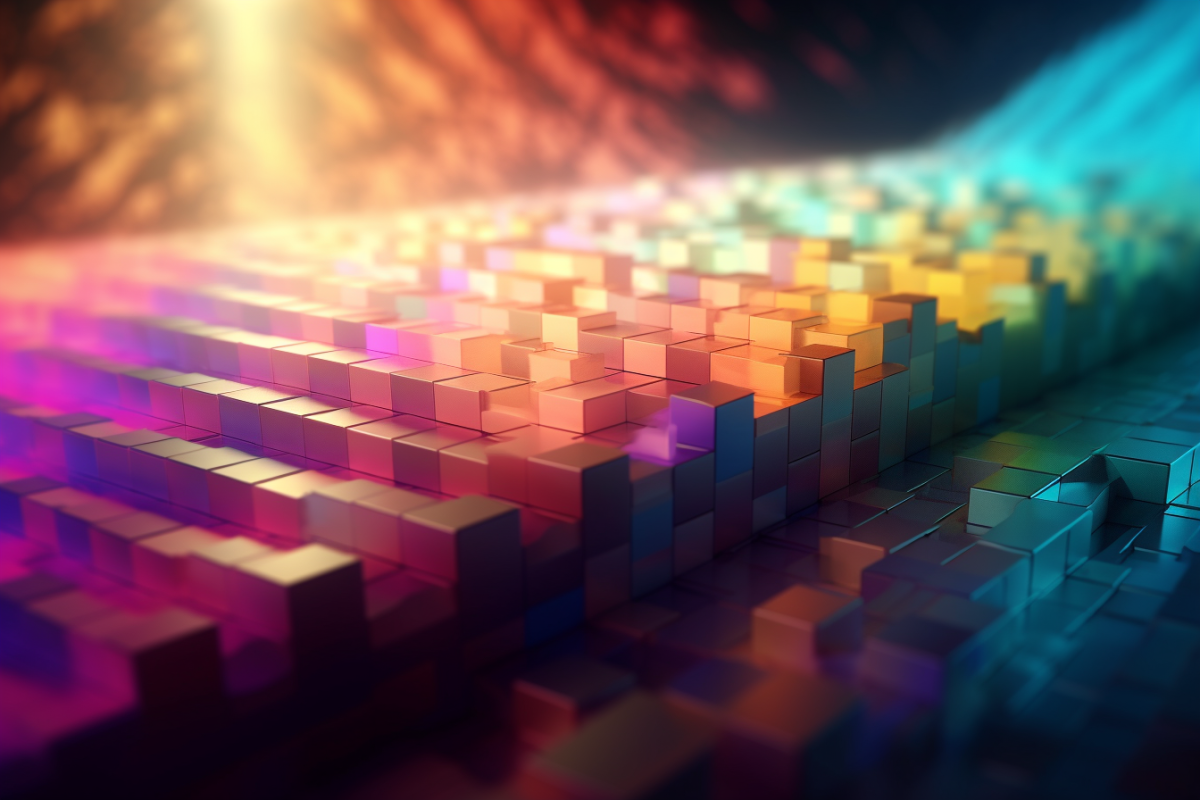
In our previous blog post, we discussed the ultimate tech stack for skill identification and management, highlighting the essential technologies that drive success in this critical area. Now, let's take a closer look at how these technologies come together in a comprehensive workflow to streamline and optimize skill identification and management for modern businesses.
1. Data Collection and Preprocessing:
The first step in the skill management workflow is to collect and preprocess the necessary data. This involves gathering unstructured data from various sources, such as resumes, job descriptions, and social media profiles, as well as structured data from internal systems like HRIS and applicant tracking systems. Using Application Programming Interfaces (APIs), data can be seamlessly imported and integrated from these sources.
Natural Language Processing (NLP) tools are then used to preprocess and clean the data, removing irrelevant information and formatting the remaining data in a consistent and structured manner.
2. Skill Extraction and Analysis:
Once the data is preprocessed, NLP and Machine Learning (ML) algorithms can be used to extract and analyze skills from the structured data. These algorithms identify key skills, qualifications, and experiences within the data and map them to standardized definitions, ensuring consistency across the entire dataset.
At this stage, the relationships between skills, job roles, and industries are also analyzed using ML algorithms and graph databases. This helps to uncover patterns, trends, and connections that can inform workforce planning and employee development initiatives.
3. Skill Data Enrichment:
The skill data is further enriched by integrating external data sources, such as job boards, professional networks, and learning management systems. APIs are used to access and import this external data, which can provide valuable context for the identified skills and help to refine the skill definitions and relationships.
4. Skill Data Visualization and Reporting:
With the skill data cleaned, analyzed, and enriched, the next step is to present this information in a user-friendly format using data visualization tools. These tools enable HR professionals and business leaders to quickly identify insights and trends, allowing them to make informed decisions based on skill data.
Interactive dashboards and visualizations can be created to display skill data in various formats, such as heatmaps, scatter plots, and network graphs. These visualizations can be easily customized to meet the specific needs of the organization and support ongoing skill management efforts.
5. Continuous Monitoring and Updating:
As the job market and industry requirements evolve, it's crucial to ensure that the skill data remains up-to-date and relevant. The skill management workflow should include continuous monitoring and updating of skill data, using NLP and ML algorithms to track changes in skill definitions, requirements, and relationships.
By regularly updating the skill data, organizations can stay informed about the latest trends and shifts in the job market, enabling them to make proactive decisions about workforce planning and employee development.
Integrating cutting-edge technologies like NLP, machine learning, graph databases, APIs, and data visualization tools, the ultimate tech stack for skill identification and management can be harnessed to create a comprehensive workflow that streamlines and optimizes the entire skill management process. This powerful workflow enables businesses to effectively identify, track, and manage skills within their organization, ensuring they remain competitive and agile in the ever-evolving modern workforce.