Conceptual Semantic Search vs. Traditional Semantic Search: Why Prioritizing Similar Candidates Matters
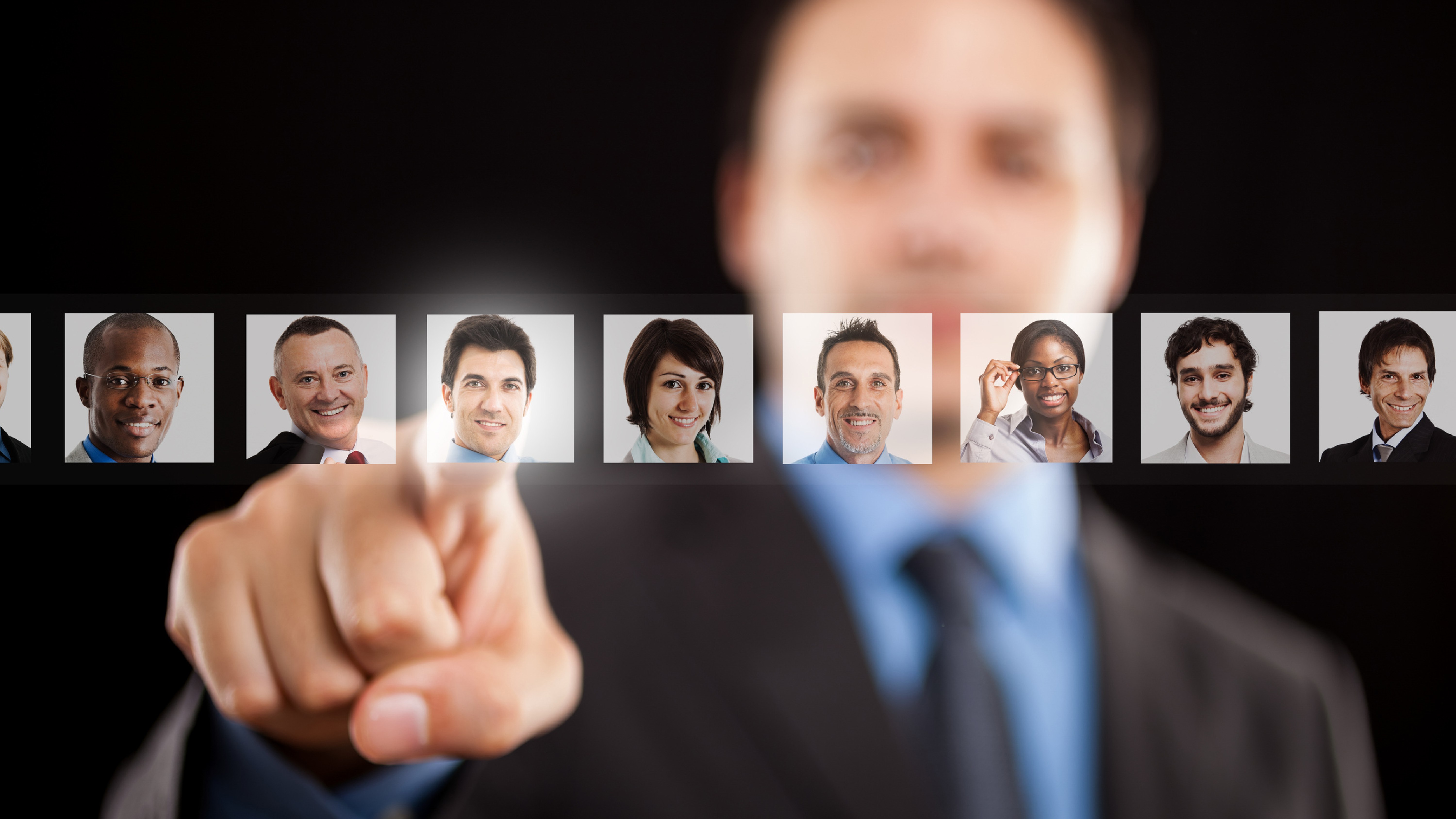
When it comes to staffing agencies, one of the biggest challenges is identifying the right candidates for a job. While traditional semantic search can help narrow down the talent pool, it often fails to prioritize candidates with similar experiences and qualifications. This is where conceptual semantic search comes in.
Conceptual semantic search uses machine learning algorithms to understand the meaning behind words and phrases, allowing it to identify candidates based on the context of their skills and experiences rather than just keyword matches. This means that even if a candidate's resume does not contain the exact keywords that a recruiter is searching for, they may still be a strong candidate if they possess the relevant skills and experiences.
By using conceptual semantic search to narrow down a talent pool, staffing agencies can save time and improve the quality of their matches. Instead of manually sifting through resumes and applications, recruiters can use candidate intelligence solutions to quickly identify candidates with the right combination of skills and experiences. This not only improves the efficiency of the recruiting process but also helps to reduce bias by ensuring that candidates are evaluated based on their qualifications rather than superficial characteristics.
Additionally, using conceptual semantic search can help identify candidates with transferable skills or experiences that may not have been apparent through traditional semantic search. This expands the potential talent pool and enables staffing agencies to tap into a wider range of qualified candidates.
Overall, the advantages of narrowing down a talent pool using conceptual semantic search include improved efficiency, reduced bias, and access to a wider range of qualified candidates. By embracing the power of machine learning and candidate intelligence, staffing agencies can improve their recruitment processes and ultimately, provide better matches for their clients.
Sources:
Raghavan, V., & Garcia-Molina, H. (2001). Concept search on the web. ACM Transactions on Information Systems, 19(1), 1-37.
Shen, D., Wang, J., & Zhang, J. (2014). Concept-based document relevance feedback using interactive genetic algorithm. Information Processing & Management, 50(1), 124-143.
Wu, F., Wu, Y., Jiang, J., & Xu, J. (2016). A survey of semantic search approaches. Journal of Informetrics, 10(4), 917-940.